Data Enrichment: Elevating Efficiency in AI/ML Training Workflows
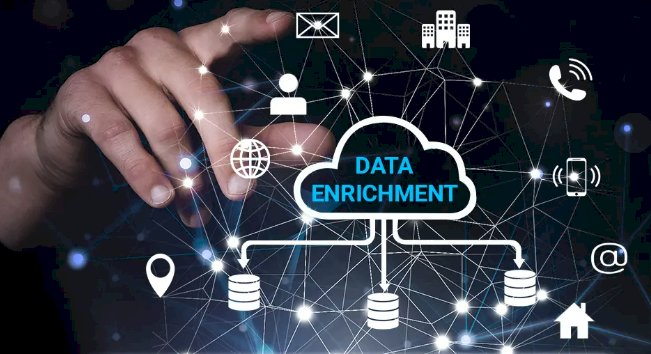
When talking about artificial intelligence (AI) and machine learning (ML), the phrase “Garbage In, Garbage Out” (GIGO) stands as a powerful reminder of the critical role input data quality plays in shaping outcomes. The effectiveness of machine learning and deep learning models is intricately tied to the quality of their training data. When the foundational data contains bias, incompleteness, or errors, it leads to unreliable and potentially skewed outcomes.
To avert the pitfalls of GIGO, meticulous measures such as data cleaning, enrichment, or augmentation are imperative. As we embark on the journey toward AI excellence, the core principle remains clear: commitment to ensuring that input data is enriched and high quality is paramount.
What good quality training data looks like?
It is:
1. Relevant
- Definition: Dataset includes only attributes providing meaningful information.
- Importance: Requires domain knowledge for feature selection.
- Impact: Enhances model focus and prevents distraction from irrelevant features.
2. Consistent
- Definition: Similar attribute values correspond consistently to similar labels.
- Importance: Maintains dataset integrity for reliable associations.
- Impact: Facilitates smooth model training with predictable relationships.
3. Uniform
- Definition: Comparable values across all data points, minimizing outliers.
- Importance: Reduces noise and ensures model stability.
- Impact: Promotes stable learning patterns for effective generalization.
4. Comprehensive
- Definition: The dataset includes enough features to address various scenarios.
- Importance: Provides a holistic understanding of robust models.
- Impact: Enables effective handling of diverse real-world challenges.
Factors affecting training data quality
Several factors influence the quality of training datasets, impacting the model’s performance and generalization. Understanding these is crucial for developing strategies to enhance dataset quality. Here are some of the key aspects that can affect the quality of training datasets:
1. Data source selection
Data collection methods
3. Data volume and diversity
4. Data preprocessing technique
5. Labeling accuracy
6. Data bias
7. Domain-specific challenges
Addressing the challenges of low-quality data with enrichment
Raw data, while essential, often lacks completeness or may not capture the full context needed for effective machine learning. Enter data enrichment – the process of enhancing and expanding the raw dataset to improve its quality. This helps in creating detailed training datasets that provide comprehensive information to AI models. Failure to enrich data properly can compromise the dataset’s quality, thereby constraining the model’s understanding and leading to inaccurate predictions.