From Data Silos to Dynamic Responses: Leveraging RAG for Reliable GenAI
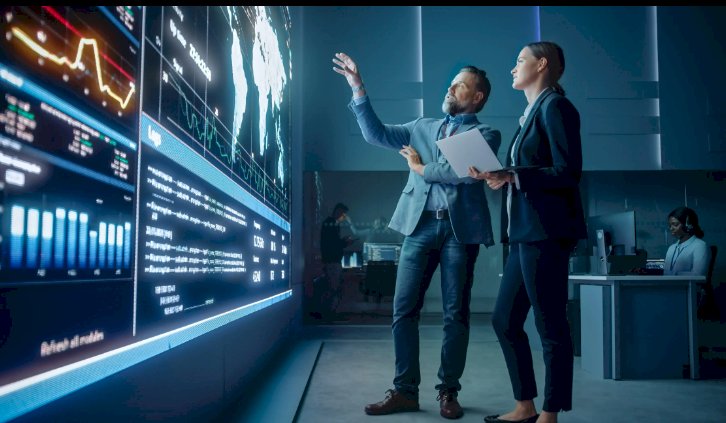
The world of GenAI is filled with all kinds of possibilities. From making rhymes to generating code, the LLMs have come a long way. The question at hand is not about whether GenAI can deliver accurate and verifiable information but whether we can trust GenAI to do so. This is the point where Retrieval-Augmented Generation (RAG) comes into play.
Although the new generation of LLMs do a good job of creating comprehensible stories, their knowledge base is closed. Plus, they are ignorant about topics they were not trained on. This may result in a disproportionate number of errors and responses containing old information. Furthermore, complex queries may cause LLMs to respond in an incoherent manner.
This case study demonstrates the tangible benefits of RAG in real-world applications. Integrating RAG into GenAI systems like chatbots helps them to become more dependable and enhance efficiency, which, in turn, improves the customer experience.
With RAG technology, GenAI stands at the threshold of abandoning these limitations and entering an era of dependability and confidence. With the advent of RAG, we will see a generation of AI apps that are no longer only creative but also reliable.